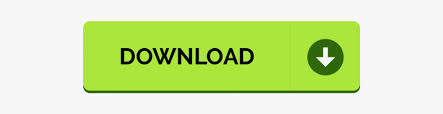

Let’s start introducing a basic regression of the logarithm of the wage(ln_wage) on age(age), job tenure(tenure) and race(race). With the –regress- command, Stata performs an OLS regression where the first variable listed is the dependent one and those that follows are regressors or independent variables. I am not going to discuss panel data now but it is good if we start to know the database that I will use in the next posts to introduce panel data. If you want to describe data, type describe and you will see that this is a panel data of women of 14-26 years providing information regarding their race, marital status, educational attainment and employment.
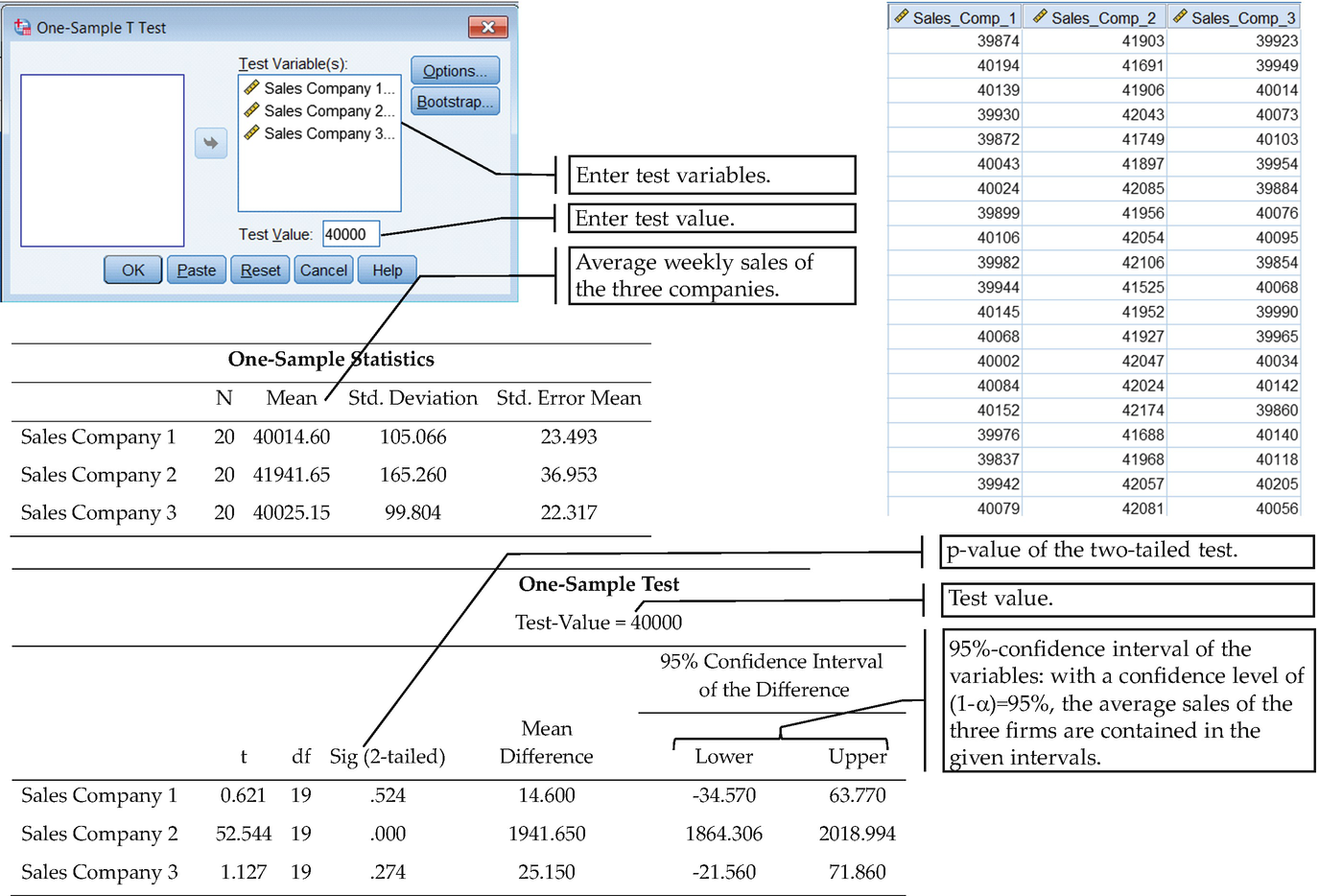
In order to investigate some interesting relations we must abandon our auto.dta dataset and use a subsample of Young Women in 1968 from the National Longitudinal Survey(nlswork) available by typing: An incorrect functional form can lead to biased coefficients, thus it is extremely important to choose the right one. You should choose the model with the higher coefficient of determination in this case. We can try to follow the literature on the topic and use the common sense or decide to compare the R-Squared of each form as long as the dependent variables are the same. Given that sometimes we have huge amounts of data, this procedure becomes unfeasible. If the scatterplot exhibits a non-linear relationship, then we should not use the lin-lin model. My personal opinion is that we should choose the model based upon examining the scatterplots of the dependent variable and each independent variable. The marginal effect depends on the other regressor.
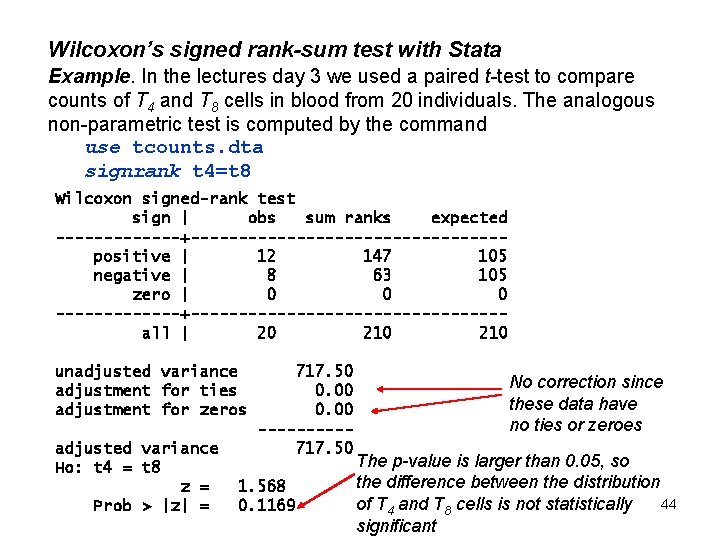
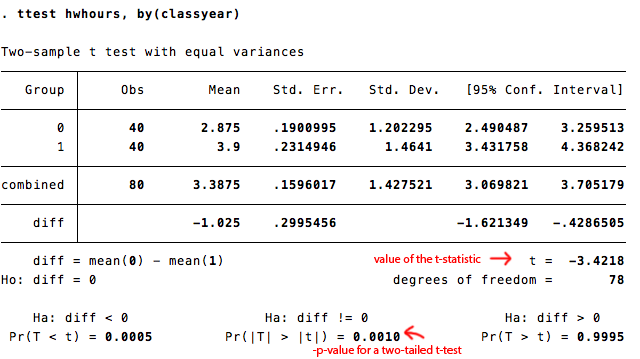
In this model, the β1 coefficient can be interpreted as the marginal effect age has on wage if race=0. If we want to compute an interaction term between two independent variables to explore if there is a relation we can write: This model is usually described with graphs of trajectory. The marginal effect of age on wage depends now on the values that age takes. In this model, one of the independent variables is included in its square as well as linear terms. Beta can be interpreted as the unitary variation of write score respect to the relative variation of the math score. The regressor is log transformed while the dependent variable is linear. This model is the opposite of the previous one. Since this is just an ordinary least squares regression, we can easily interpret a regression coefficient, say β1, as the expected change in log of write with respect to a one-unit increase in math holding all other variables at any fixed value. Very often, a linear relationship is hypothesized between a log transformed outcome variable and a group of predictor linear variables likes: Indeed, beta is the percent variation of lwrite associated with a 1% variation of lmath. In this model, the beta coefficient may be interpreted as elasticity of lwrite respect to lmath. In our example, I have log transformed a hypothetical writing and math scores test. In this model, both the dependent and independent variables are logarithmic. However, if we abandon this hypothesis, we can study several useful models whose coefficients have different interpretations. One of the assumptions of the OLS model is linearity of variables. I am only going to discuss some modeling strategy. I am sorry but I am not going to give you a theoretical explanation of what we are doing so, if you are not familiar with the argument yet, I suggest you to check The Econometrics’ Bible: Wooldridge. Today we are ready to start with the grass-roots econometric tool: Ordinary Least Square (OLS) Regression! We will revise several commands that I already described in previous posts so, in case you missed them, you have the opportunity to review them again.
#T test stata how to#
Have you ever wondered how to make regressions and test them using Stata? If the answer is Yes, read below…
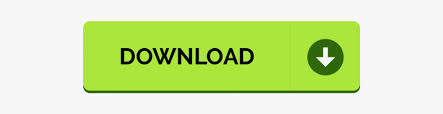